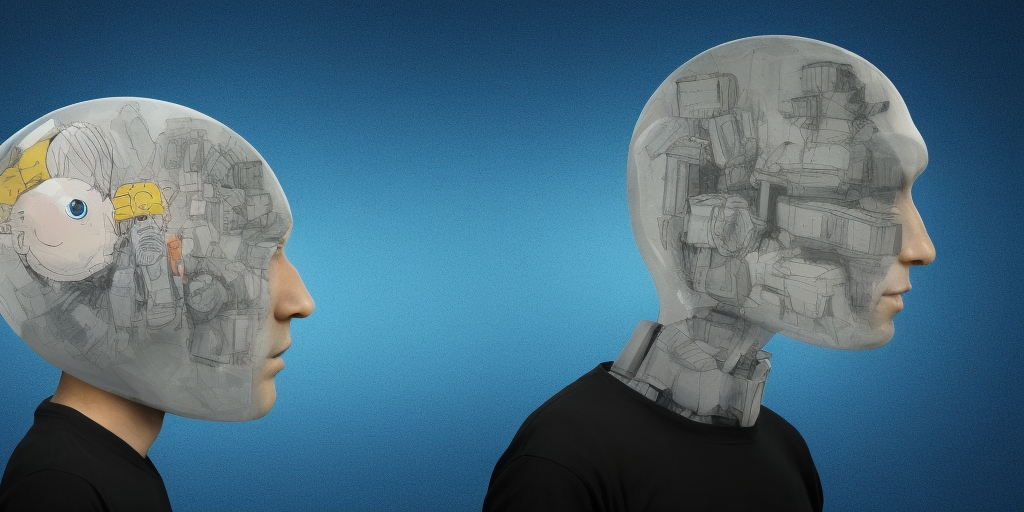
This post is a more general discussion about ChatGPT and related systems – it’s important to cut through the hype surrounding LLMs and absorb the sober scientific and cultural perspectives and questions around these systems and their capabilities. The impacts of these systems for knowledge work and creative work is going to be huge in the near future – so time to start understanding their context and implications.
S. Wolfram : What Is ChatGPT Doing … and Why Does It Work?
This is an excellent technical discussion about how Neural Nets (NNs) work, with interesting questions about the internal ‘black box’ goings-on – that are in general quite inscrutable. Wolfram is arguing for a rigorous scientific understanding of NNs, as they seem principally to have arisen as engineering exercises – things that work, but no-one really understands exactly why (‘lore’ in Wolfram’s estimation). This is a sharp counterpoint to the feuilleton hype about AI (which is, really, ‘Machine Learning’, or ‘Machine Representation’, as it is not ‘truly’ intelligent or aware). He makes interesting points about interfacing something like ChatGPT with Wolfram Alpha, which is a kind of computational knowledge engine, and argues convincingly that an interface between the two systems could solve many of the factual errors confabulated by the LLM, and provide something much more powerful in combination: a system that is ‘factually’ connected to the ‘world’ – and perhaps even capable of causal inference as a result.
The discussion touches upon several interesting philosophical/theoretical areas concerning the construction and emergence of language and discourse.
Human language—and the processes of thinking involved in generating it—have always seemed to represent a kind of pinnacle of complexity. And indeed it’s seemed somewhat remarkable that human brains—with their network of a “mere” 100 billion or so neurons (and maybe 100 trillion connections) could be responsible for it. Perhaps, one might have imagined, there’s something more to brains than their networks of neurons—like some new layer of undiscovered physics. But now with ChatGPT we’ve got an important new piece of information: we know that a pure, artificial neural network with about as many connections as brains have neurons is capable of doing a surprisingly good job of generating human language. (Wolfram, 2023)
Of great interest to me is the possibility of what one might call ’empirical semiotics’ or ‘computational semiotics’ – where semiotic generation and analysis (semiosis) could be underpinned by computational forms of emergence, categorisation and logic.
The success of ChatGPT is, I think, giving us evidence of a fundamental and important piece of science: it’s suggesting that we can expect there to be major new “laws of language”—and effectively “laws of thought”—out there to discover. In ChatGPT—built as it is as a neural net—those laws are at best implicit. But if we could somehow make the laws explicit, there’s the potential to do the kinds of things ChatGPT does in vastly more direct, efficient—and transparent—ways. (Wolfram, 2023)
Presumably many of these ‘laws’ are already uncovered or at least hinted-at by research in NLP and computational language models – but it appears very much a contentious field – especially with regard to what any formulation of what ‘intelligence’ is.
If there is one constant in the field of artificial intelligence it is exaggeration: There is always breathless hype and scornful naysaying. It is helpful to occasionally take stock of where we stand. (Browning & LeCun, 2022b)
To me it seems important to understand LLM cognates and extensions in multimodal systems – that intelligent systems can draw inferences across visual, audial, somatic and other sensory modalities beyond the textual and linguistic (Browning & LeCun, 2022a).
The underlying problem isn’t the AI. The problem is the limited nature of language. Once we abandon old assumptions about the connection between thought and language, it is clear that these systems are doomed to a shallow understanding that will never approximate the full-bodied thinking we see in humans. In short, despite being among the most impressive AI systems on the planet, these AI systems will never be much like us.(Browning & LeCun, 2022a).
The field of semiotics has a significant body of work covering these differing/interdependent signifying regimes – but not a great deal that is computationally reducible, as it has been more in the form of ‘literary criticism’ or humanities ‘theory’ (including in my own research background). This is clearly inadequate for a scientific approach as it is far too qualitative – more ‘top down’ than ‘bottom up’ – reminiscent of debates concerning symbolic reasoning vs (what might be termed) ’emergent’ reasoning. However, there are examples in the work of C.S. Peirce, M.A.K. Halliday and others that may be useful for thinking about this domain. In the area of cognitive neuroscience/neuroanthropology I immediately think of the work of T. Deacon, A. Damasio, D. Deutsch, J. Hawkins and others that synthesise this inter-disciplinary domain of knowledge into useful ways of thinking about what might constitute an intelligent system and how it might emerge.
For non-specialists like me there is much to absorb – that can inform ways of critically engaging with this novel technology.
It is terribly important to not be naive about this stuff (AI), as it will (and has already) have transformational impacts upon personhood, economic, political and natural systems, for good and for bad. It is hard to imagine a future when a self-aware, agentive machine intelligence is more than a science ‘fiction.’ It sounds absurd, but perhaps it isn’t.
Imagine a world where people’s online images, text, music, voice recordings, videos, and code get gathered largely without consent to train AI models, and sold back to them for $10 a month. We’re already there but imagine something beyond that – and assume it’s incredible…
…Here’s a thought experiment: imagine an AGI system that advises taxing billionaires at a rate of 95 percent and redistributing their wealth for the benefit of humanity. Will it ever be hooked into the banking system to effect its recommended changes? No, it will not. Will those minding the AGI actually carry out those orders? Again, no.
No one with wealth and power is going to cede authority to software, or allow it to take away even some of their wealth and power, no matter how “smart” it is. No VIP wants AGI dictating their diminishment. And any AGI that gives primarily the powerful and wealthy more power and wealth, or maintains the status quo, is not quite what we’d describe as a technology that, as OpenAI puts it, benefits all of humanity. (Claburn, T. , The Inquirer, 2023)
We don’t have a good definition of intelligence – so it seems best to define it operationally (as Friston et. al. 2022 does). At this stage the take-away is that LLM’s are clearly what the label says: they are language models, not artificial intelligences – they are, literally, Rhetorical Devices.
LLMs statistically parameterise a huge amount of ‘knowledge’ about linguistic representations of the world – based upon their massive set of ‘training’ data. These terms are information, signs, similes, metaphors, metonyms, synedoches – abstractions that can exhibit indefiniteness: degrees of epistemic and ontic undecidability or infinite regression. Uncertainty.
LLMs seem to respond dialogically, perhaps following chains of reasoning akin to the vectors in ‘meaning space’ that Wolfram discusses (’embeddings’ – examples of t-SNE or word2vec dimensional reduction plots). These dialogues can also be guided by user interaction via the Chatbot query interface through ‘chain-of-thought’ reasoning – which demonstrably improves the model performance (even, it seems, when the model performs what might be the equivalent of ‘self-talk’).
Larger models seem to improve inferential reasoning – yet presumably there will be drawbacks or limits in a scale-only approach. Not least amongst these being the prodigious amounts of compute required, and their concomitant use of electricity and consequent carbon-impacts.
Are they ’emulations’ or ‘simulations’? What would this distinction imply?*** To me, it indicates that it (an LLM) is a map, not an actor; a palimpsest, not an agent.
A counterpoint.
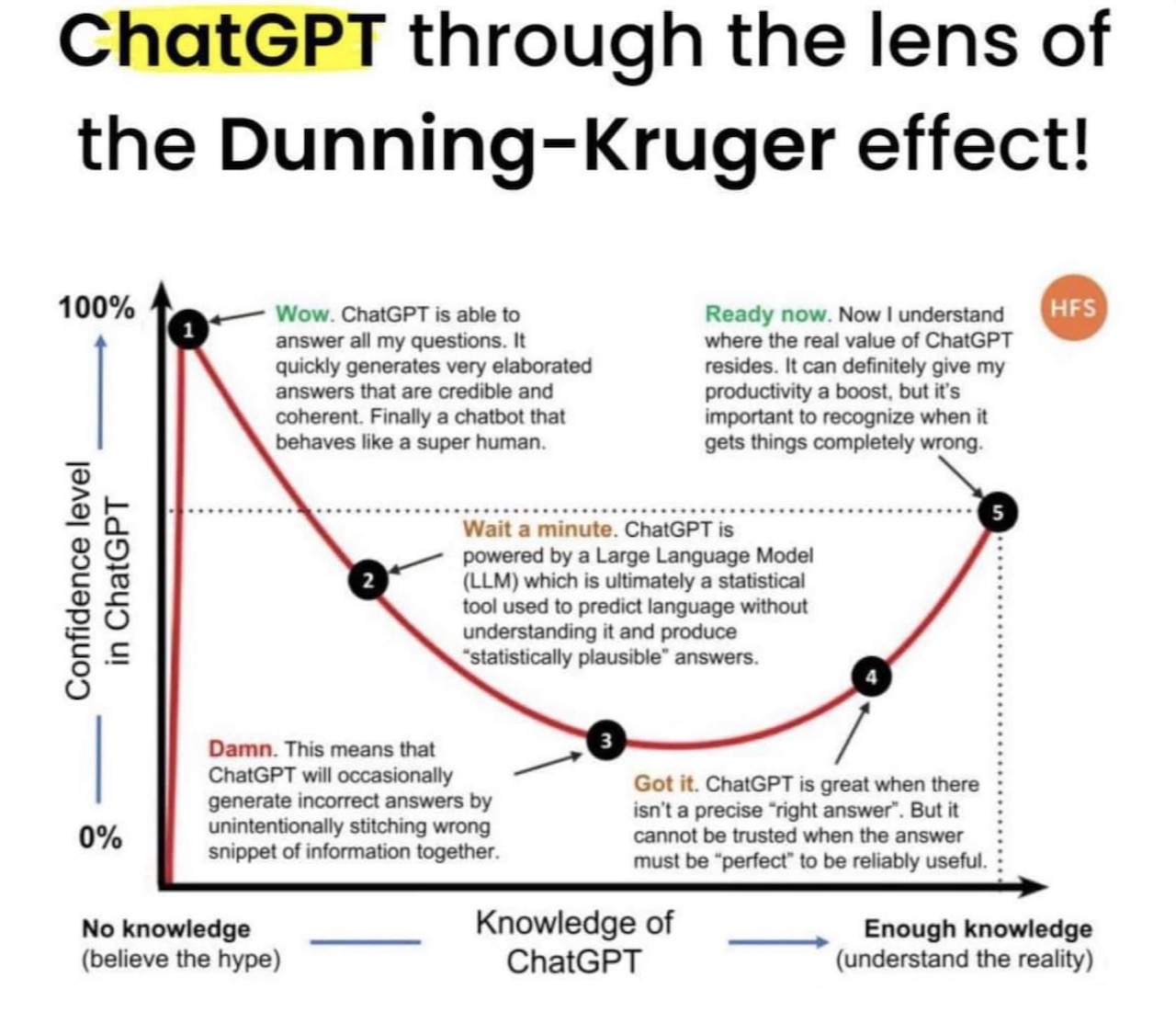
*ChatGPT apparently implements this type of response training interface – thumbs-up/thumbs-down.
***Thanks to my colleague P. Bourke for drawing this distinction to my attention.
References
Altman, S., n.d. Planning for AGI and beyond [WWW Document]. OpenAI. URL https://openai.com/blog/planning-for-agi-and-beyond#SamAltman (accessed 3.10.23).
Ananthaswamy, A., 2023. In AI, is bigger always better? Nature 615, 202–205. https://doi.org/10.1038/d41586-023-00641-w
Browning, Jacob, and Yann LeCun. “What AI Can Tell Us About Intelligence,” June 16, 2022. https://www.noemamag.com/what-ai-can-tell-us-about-intelligence.
Browning, Jacob. “AI And The Limits Of Language,” August 23, 2022. https://www.noemamag.com/ai-and-the-limits-of-language.
Claburn, T., n.d. OpenAI CEO heralds AGI no one in their right mind would want [WWW Document]. URL https://www.theregister.com/2023/02/27/openai_ceo_agi/ (accessed 3.10.23).
Daull, Xavier, Patrice Bellot, Emmanuel Bruno, Vincent Martin, and Elisabeth Murisasco. “Complex QA and Language Models Hybrid Architectures, Survey.” arXiv, February 17, 2023. http://arxiv.org/abs/2302.09051.
Deacon, Terrence W. Incomplete Nature: How Mind Emerged from Matter. WW Norton & Company, 2011.Deacon, Terrence W. The Symbolic Species: The Co-Evolution of Language and the Brain. WW Norton & Company, 1998. ISBN:9780393049916
Dennett, D.C. Consciousness Explained. Little, Brown, 2017. ISBN: 0-316-18065-3
Deutsch, David. The Beginning of Infinity: Explanations That Transform The World. Penguin UK, 2011. ISBN: 9780140278163
Friston, Karl J, Maxwell J D Ramstead, Alex B Kiefer, Alexander Tschantz, Christopher L Buckley, Mahault Albarracin, Riddhi J Pitliya, et al. “Designing Ecosystems of Intelligence from First Principles,” December 2022. https://doi.org/10.48550/arXiv.2212.01354.
Halliday, Michael Alexander Kirkwood. Language as Social Semiotic. London Arnold, 1978. ISBN:9780713159677
Hawkins, Jeff. A Thousand Brains: A New Theory of Intelligence. Hachette UK, 2021. https://doi.org/10.26613/esic.6.1.282
Huang, Jiaxin, Shixiang Shane Gu, Le Hou, Yuexin Wu, Xuezhi Wang, Hongkun Yu, and Jiawei Han. “Large Language Models Can Self-Improve.” arXiv, October 25, 2022. http://arxiv.org/abs/2210.11610.
Ouyang, Long, Jeff Wu, Xu Jiang, Diogo Almeida, Carroll L. Wainwright, Pamela Mishkin, Chong Zhang, et al. “Training Language Models to Follow Instructions with Human Feedback.” arXiv, March 4, 2022. http://arxiv.org/abs/2203.02155.
Pearl, J., Mackenzie, D., 2018. The Book of Why: The New Science of Cause and Effect. Penguin UK. ISBN: 9780141982410
Savage, N., 2023. Why artificial intelligence needs to understand consequences. Nature. https://doi.org/10.1038/d41586-023-00577-1
Tanaka-Ishii, K., 2010. Semiotics of Programming. Cambridge University Press. ISBN:9780521516556
Tenachi, W., Ibata, R., Diakogiannis, F.I., 2023. Deep symbolic regression for physics guided by units constraints: toward the automated discovery of physical laws. https://doi.org/10.48550/arXiv.2303.03192
Massimini, M., Tononi, G., 2018. Sizing Up Consciousness: Towards an Objective Measure of the Capacity for Experience. Oxford University Press. ISBN:9780198728443
Wolfram, Stephen. “What Is ChatGPT Doing … and Why Does It Work?,” February 14, 2023. https://writings.stephenwolfram.com/2023/02/what-is-chatgpt-doing-and-why-does-it-work/.